Social Network Analysis in the area of conflict between being analytic &Â considering educational research
Discussing assets and drawbacks of learning analytics (LA) and social network analysis (SNA) in particular leads to discussing the objectivity of data collection. Applying these methods not only enables live-data tracking and analysis (as opposed to self-reported pre-/post-course surveys) but the objective analysis of learner’s traces in the learning environment (though this does not account for a complete picture of the learning which takes place). However, the potential stays untapped if underlying pedagogical and epistemological assumptions are not taken into account.
Gašević, D., Dawson, S., Siemens, G. (2015) point this out as they claim LA to connect existing research on learning, teaching, educational research and practice. They bemoan missing studies which evaluate the concept of the established lead indicators of LA. Three major themes in their paper are the detailed description of how tools are used, looking beyond frequency of activity and time spent on task by analyzing individual learning strategies and products  and the connection of internal and external conditions for data. Within this context the base for effective LA visualizations lies in the consideration of instructional, learning and sense-making benefits – foundations in distributed cognition and self-regulated learning being a potential direction of future research.
“The Epistemology–Assessment–Pedagogy triad”
Knight, S. et al. (2014) identify LA as implicitly or explicitly promoting particular assessment regimes in the epistemology, assessment and pedagogy triad. A wonderful, more detailed reflection on this triad in connection to learning analytics can be found here (authored by Classroomaid). Suthers, D. D. & Verbert, K. (2013) define LA as the “middle space” between learning and analytics. In their paper they elaborate three main themes for future research in the field of LA: “the middle space” (focus on the intersection between learning and analytics, avoiding the emphasis of one of these themes), “productive multivocality” (facing the challenge of unifying a multifaceted research field by focusing on analyzing a common data ground) and “the old and the new” (enhancing learning as a century-old idea that is continuously accompanied by new tools) (pp1). Given the rich online learning landscape, clustering learning environments can be the first step of detecting characteristics, underlying epistemology-assessment-pedagogy beliefs and thus identifying the appropriate measures of learning analytics.
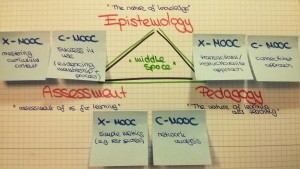
For example Rodriguez (2012) classified MOOCs as either c-MOOCs (following the connectivist tradition) or x-MOOCs (“AI-Standford like courses”, following the cognitive-behaviorist tradition). It is important to note here, that the term “x-MOOCs” was not coined by Rodriguez, but Liyanagunawardena, T., et al. (2013) establish ties to Daniel (2012), where they detected similar definitions and thus combined both papers. For his classification Rogriguez used Anderson & Dron’s (2011) paper on “Three Generations of Distance Education Pedagogy” where they coin three DE pedagogy concepts. Bringing the triad back into focus: it’s the pedagogy concept that supports his classification – differentiating between connectivistic and cognitive-behavioristic pedagogy.
The implication of this classification is a different view on teaching, social and cognitive presence in the online learning environment. This view needs to be considered when analyzing the underlying epistemological concept and the assessment formats. Besides common features, this relates especially to the role of course instructors, the definition of openness (access vs. openness to personalized learning), connectedness and guidance. Knowledge is either generative (c-MOOC) or declarative (x-MOOC). By saying so, without a coherent triad the best assessment strategy does not tackle the real learning happening. Furthermore, the triad can be used to continuously challenge the assumptions of each corner.
Active Learning – an example for effective college classroom practice
One example of this triangular interplay is active learning. Grunspan et al. (2014) mention the effectiveness of active learning in college classrooms and as a result they explore this practice with the support of Social Network Analysis. However, the triad does not implicitly determine direction and interdependencies. Critical reflection of all intersections is necessary. This exemplifies the importance of meta studies, e.g. “What makes great teaching? Review of the underpinning research” by Robert Coe, Cesare Aloisi, Steve Higgins and Lee Elliot Major (2014). A call for a constant challenge of learning assumptions, the participation in the on-going research process and the relation and integration of own research efforts go hand in hand with the importance of comparing different underlying concepts and critically questioning if researchers are particularly considering the same concepts. As one example of this meta study, active learning and its outcomes for the learning progress seem to contradict Grundspan et. al. By relating to a learning pyramid, Coe et al. argue that memory and remembering is not evidently based on being better when participating actively or passively (“Ensure learners are always active, rather than listening passively, if you want them to remember (p24)). Simply the different level of complexity of both concepts (active learning and active/passive listening in relation to memorizing) discloses that we are not exposed to the same ideas here. However, these approaches seem to be connected and point in opposing directions of sound research evidence. When validating and examining existing research, it becomes more and more important to nail the underlying assumptions and research questions to create reliable conclusions for further research.
Six different case studies: Learning design can influence learner’s decision
The course presents six different case studies which emphasize the claim that learning design can influence learner’s decision. By doing so, the above triad is underpinned again – not only focusing on practical applications but also giving a direction for future research approaches that critically question underlying concepts of epistemology, pedagogy and assessment.
1. Instructor-centered network: Bringing together SNA and learning design [Lockyer, L., Heathcote, E., & Dawson, S. (2013)]
Lockyer et al. explore how the framework for the interpretation of LA might lie in the learning design. By using case-based learning they examine a concept of checkpoint and process analytics to analyse learning design embedded in a context, in real-time and behavior-based (a more narrowed-down application of LA). Hence, learning design and analytic design are connected to support learning and teaching decisions. They also propose different directions for future research including “engaging teachers and students in understanding and using visual patterns of interaction as a means to encourage learning activity; scaling up to larger numbers of classes, providing a base for comparing statistically the observed to expected analytics of behaviors and interactions; and using results to provide meaningful feedback to teachers on how their learning design is meeting their pedagogical goal and to assist them in decisions around design and pedagogical change in real time.” (p1455)
2. Sense of community [Dawson, S. (2008)]
The research question in this study is “Is the composition of social networks evolving from a unit discussion forum related to the sense of community experienced among the student cohort?” (p226). In general, this deals with the question of belonging vs. isolation, or better: with the extend to which learners benefit educationally from belonging and how this can operate as a predictor for students success (as social integration is strongest predictor for retention and completing university degree). The underlying educational concept is community-centered teaching practise, based on social-constructivistic ideas of Dewey and Vygotsky. The novelty with this study is that self-reported surveys are not the only data source anymore. It is a mixed method approach used here which focuses on quantitative (Classroom Community Scale, SNA centrality measures) and qualitative measures (discussion forum content, student interviews). As a result, Dawson found a association between the network position and the sense of community, in detail a positive association with closeness and degree centrality; a negative with betweenness centrality (dilemma of brokerage). In addition, pre-existing external social networks influence the type of support/information required. Concerning future research Dawson points towards the investigation of the relation between social networks and other measures having an influence on the learning environment such as pedagogy, practitioner personality and cohort demographic profiles.
3. Network brokers associated with achievement and creativity [Dawson, S., Tan, J. P. L., & McWilliam, E. (2011)]
Dawson, S. et al. discuss the correlation of cognitive playfulness to the network position where degree and betweenness centrality are oppositional to closeness (as they are positive indicators of a learner’s creative capacity) . By answering their research questions “What is the relationship between a student’s social network position and perceived creative capacity? To what extent do discussion forum mediated social networks allow for the identification and development of student creativity?” they claim that SNA can provide insight in the creativity of students as well as a tool for instructors to monitor the learner’s creative capacity level. The individual’s self-reported creativity score thus corresponds with the overall social network position. Creativity is perceived as highly valued graduate asset.
4. SNA for understanding and predicting academic performance [Gašević, D., Zouaq, A., Jenzen, R. (2013)]
Gašević, D. et al. studied cross-class networks and the importance of weak ties by considering the relationship between academic performance and socal ties. The base for this study is social capital and network learning research. Two hypothesis where investigated: (1) “students’ social capital accumulated through their course progression is positively associated with their academic performance”; and (2) “students with more social capital have a significantly higher academic performance.” Based on the ideas of Vygotsky one practical implication is the conception of new social ties in each course during degree programs.
5. SNA and social presence (What is the association between network position and social presence?) [Kovanović, V., Joksimović, S., Gašević, D., Hatala, M.]
This study focuses on the Community of Inquiry model, specifically on the social presence as one contributor to educational experience. Social presence consists of three parts, namely affectivity and expression, interactivity and open communication and cohesiveness. By analyzing the underlying social processes that contribute to the development of social capital, Kovanović, V. et al. give an insight in how affective, cohesive and interactive facets of social presence significantly predict the network centrality measures commonly used for measurement of social capital. Social constructivist pedagogies and the shift towards collaborative learning can be seen as underlying educational concepts. The research question “What is the relationship between the students’ social capital, as captured by social network centrality measures, and students’ social presence, as defined by the three categories in the Community of Inquiry model?” (p3) leads to the results that interactive social present is “most strongly associated with all of the network centrality measures, indicating a significant relation with the development of the students’ social capital.” In conclusion, in-degree and out-degree centrality measures were predicted by all categories of  social presence whereas betweenness centrality was predicted by interactive and affective categories.
6. SNA and understanding of MOOCS [Skrypnyk, O., Joksimović, S. Kovanović, V., Gasevic, D., Dawson, S. (2014)]
Skrypnyk, O. et al. explore the learning environment of a cMOOC to identify and understand important key actors. Although in this study the facilitators continued to occupy a central role, other actors emerged and complemented this picture. This was based on the two research questions “What is the influence of original course facilitators, course participants (ie., learners) technological affordances on information flows in different stages of a cMOOC?” and “What are the major factors that influence the formation of communities of learners within the social network developed around a cMOOC?”. As a result, types of authorities can be classified as “hyperactive aggregators” and “less visible yet influential authorities”. For the former, there might be an existing connection to natural personality traits. Another outcome is the importance of hashtags for information flow and community construction within a more learner-centered environment supported by software.
Application in Gephi and Tableau
By combining Gephi and Tableau I created a dashboard, where it is possible to see different ways of visualizing the same data source. Please click the image to enlarge it and see descriptions for the single sheets on the dashboard. As my first attempt, this dashboard shall illustrate how conclusions could be drawn from one single glance.
For example, the network analysis with Gephi detects the network structure and reveals node 3 as a central key actor when it comes to the measure of degree (top right). 9, 10 and 11 follow with some distance. The same pattern can be detected in the top left visualization, where size represents degree as well, but the color reveals betweenness. We can conclude, that degree and betweenness are correlated, as a decreasing quantity of connected nodes goes hand in hand with a decreasing betweenness (not surprisingly, but due to the image more visual). The Degree sheet down left specifies in- and out-degree values – again we see the network key players 3, 9, 10, 11 but here we can specify their communication patterns. Whereas 3 has the highest out-degree but the smallest in-degree, it’s actor 11 that has the highest in-degree and the smallest out-degree. Dependent on the underlying question, we could draw better conclusions at a glance from this data.
Still, there are some open questions regarding Gephi from my last post but I found a nice point of departure from @Edu_k ‘s blog post on Gephi Layouts.
Resources
Anderson, T. and Dron, J. (2011). Three Generations of Distance Education Pedagogy,International Review of Research in Open and Distance Learning, Volume 12, Number 3. Retrieved from http://www.irrodl.org/index.php/irrodl/article/view/890/1663
Classroomaid (2014-11-14). Our Learning Analytics are Our Pedagogy, Are They? (#xAPI, #dalmooc), retrieved on 2014/10/23 from http://classroom-aid.com/2014/11/14/our-learning-analytics-are-our-pedagogy-are-they-xapi-dalmooc/
Daniel, J 2012. Making Sense of MOOCs: Musings in a Maze of Myth, Paradox and Possibility.Journal of Interactive Media in Education 2012(3):18, DOI: http://dx.doi.org/10.5334/2012-18
Dawson, S. (2008). A study of the relationship between student social networks and sense of community. Educational Technology & Society, 11(3), 224–238 (full text).
Dawson, S., Tan, J. P. L., & McWilliam, E. (2011). Measuring creative potential: Using social network analysis to monitor a learners’ creative capacity. Australasian Journal of Educational Technology, 27(6), 924-942 (full text).
Edu_k (2014/11/14). Social capital in SNA for LA – too much focus on individuals at a cost of the group, retrieved on 2014/11/24 from http://nauczanki.wordpress.com/2014/11/14/social-capital-in-sna-for-la-too-much-focus-on-individuals-at-a-cost-of-the-group/
Gašević, D., Dawson, S., Siemens, G. (2015). Let’s not forget: Learning analytics are about learning. TechTrends (in press),http://www.sfu.ca/~dgasevic/papers_shared/techtrends2015.pdf
Gašević, D., Zouaq, A., Jenzen, R. (2013). Choose your Classmates, your GPA is at Stake!’ The Association of Cross-Class Social Ties and Academic Performance. American Behavioral Scientist, 57(10), 1459-1478. doi: 10.1177/0002764213479362 (full text).
Grunspan, D. Z., Wiggins, B. L., & Goodreau, S. M. (2014). Understanding Classrooms through Social Network Analysis: A Primer for Social Network Analysis in Education Research. CBE-Life Sciences Education, 13(2), 167–178. doi:10.1187/cbe.13-08-0162 (full text)
Knight, Simon; Buckingham Shum, Simon and Littleton, Karen (2014). Epistemology, assessment, pedagogy:
where learning meets analytics in the middle space. Journal of Learning Analytics (In press).
Kovanović, V., Joksimović, S., Gašević, D., Hatala, M., “What is the source of social capital? The association between social network position and social presence in communities of inquiry,” In Proceedings of 7thInternational Conference on Educational Data Mining – Workshops, London, UK, 2014 (full text).
Liyanagunawardena, T., Adams, A., & Williams, S. (2013). MOOCs: A systematic study of the published literature 2008-2012. The International Review Of Research In Open And Distance Learning, 14(3), 202-227. Retrieved from http://www.irrodl.org/index.php/irrodl/article/view/1455/2531
Lockyer, L., Heathcote, E., & Dawson, S. (2013). Informing pedagogical action: Aligning learning analytics with learning design. American Behavioral Scientist, 57(10), 1439-1459, doi:10.1177/0002764213479367 (full text).
Rodriguez, C. O. (2012). MOOCs and the AI-Stanford like courses: Two successful and distinct course formats for massive open online courses. European Journal of Open, Distance and E-Learning. Retrieved from http://www.eurodl.org/?p=Special&sp=init2&article=516
Suthers, D. D., & Verbert, K. (2013). Learning analytics as a “middle space.” In Proceedings of the Third International Conference on Learning Analytics and Knowledge (pp. 1–4). New York, NY, USA: ACM. doi:10.1145/2460296.2460298
Skrypnyk, O., Joksimović, S. Kovanović, V., Gasevic, D., Dawson, S. (2014). Roles of course facilitators, learners, and technology in the flow of information of a cMOOC. British Journal of Educational Technology(submitted) (full text).